Table of contents
As cities continue to evolve towards more sustainable mobility solutions, cycling is gaining importance. Bicycle-friendly cities such as Copenhagen and Utrecht demonstrate the significance of well-planned and safe infrastructure in promoting cycling. As the role of bicycles in transport planning grows, so does the need for detailed and accurate representation in transport models.
However, data on bicycle traffic is often lacking. A project in the Bordeaux Region in France addressed this issue, enhancing the informative value of traffic models concerning cycling.
“Our goal was to enhance Bordeaux Metropole’s multimodal transport model (MMM33) by improving the representation of cyclists in both route choice and mode choice,” says Bastien Guichardaz from the PTV Group team in France.
The Challenges of Bicycle Traffic Modeling
Traditionally, cyclists in traffic models have been represented with a fixed speed, often overlooking the impact of dedicated infrastructure. This simplistic approach is becoming increasingly inadequate as bicycle usage grows, particularly with the rise of e-bikes. The challenge lies in the lack of detailed data on bicycle speeds, which impacts travel time calculations – a crucial component of transportation models.
“Speed determines travel time, and travel time is the key indicator in models to compute trip destinations, mode choice, and route choice,” explains Bastien Guichardaz.
Unlike cars and public transport, which benefit from extensive datasets, cycling data has historically been scarce. The absence of enforced speed limits and the numerous factors influencing cyclist speed, such as road conditions, infrastructure, and rider experience, make it difficult to establish reliable models.
“For reliable transport models, we need reliable data,” Bastien adds.
Collecting Data for a More Accurate Model
To address these gaps, the project team recruited a panel of cyclists who provided GPS records of their bicycle trips. Participants also completed surveys detailing their personal characteristics. “This cycling data collection method enabled a comprehensive analysis of trip length, speed, and infrastructure usage,” says the PTV expert.
Key Findings and Insights
The analysis confirmed that factors like age, terrain inclination, and bicycle type significantly influence speed, aligning with existing literature. However, some unexpected insights emerged.
Bastien Guichardaz states: “We were surprised by the influence infrastructure has. The base speeds identified in our analysis were quite different from the assumptions used in models so far.”
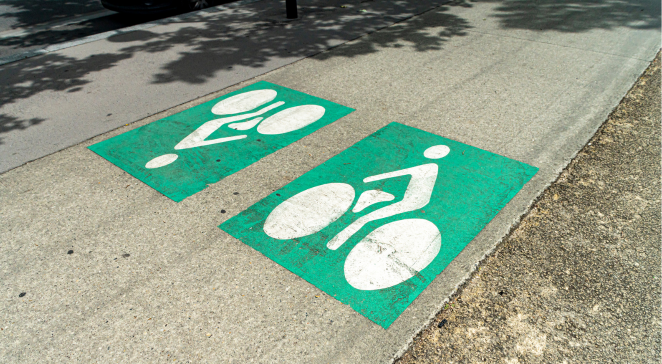
In France, cyclists are allowed to use bus lanes, for example. The study found that cyclists tend to travel faster on these shared bus lanes, despite them not being the most bike-friendly infrastructure. This trend was consistent across all cyclist experience levels.
“Our interpretation is that shared lanes provide more space and overtaking opportunities, resulting in higher speeds.”
Another major finding was that cyclists do not always choose the shortest routes. Even after accounting for adjusted speeds based on infrastructure and inclination, the actual paths taken often deviated, indicating a preference for comfort and safety over pure efficiency. This behavior was particularly evident among beginner cyclists and e-bike users, who tended to take more significant detours to use dedicated infrastructure.
“Our survey paid particular attention to e-bikes, as they reduce physical effort, increase comfort, and allow for longer trips at higher speeds,” explains Bastien Guichardaz. “Given their growing adoption in urban areas, it was essential to integrate them into the model to reflect their distinct characteristics. The collected data confirmed that e-bike users exhibit different route choices and speeds compared to conventional cyclists, reinforcing the need for differentiated modeling approaches.”
Updating Bordeaux’s Transport Model
With the collected cycling data, planners developed a specific bicycle speed model and calibrate a comfort/security bonus. Both were then integrated into Bordeaux Metropole’s multimodal transport model, enhancing the accuracy of bicycle traffic representation. By refining speed predictions and incorporating infrastructure influences, this update ensures that cycling is modeled with the same reliability as private cars and public transport.
Future Prospects for Bicycle Traffic Modeling
The enhanced model provides Bordeaux Metropole with a more robust tool for traffic planning. With improved reliability, transport planners can confidently assess the impact of new cycling infrastructure and implement policies that encourage bicycle usage. This advancement not only supports sustainable mobility goals but also enhances overall urban traffic management by integrating bicycles as a fully recognized mode of transportation.
As other cities seek to expand their cycling infrastructure, Bordeaux’s approach serves as a benchmark for leveraging data-driven insights to improve bicycle traffic modeling, ultimately fostering safer and more efficient urban mobility solutions.
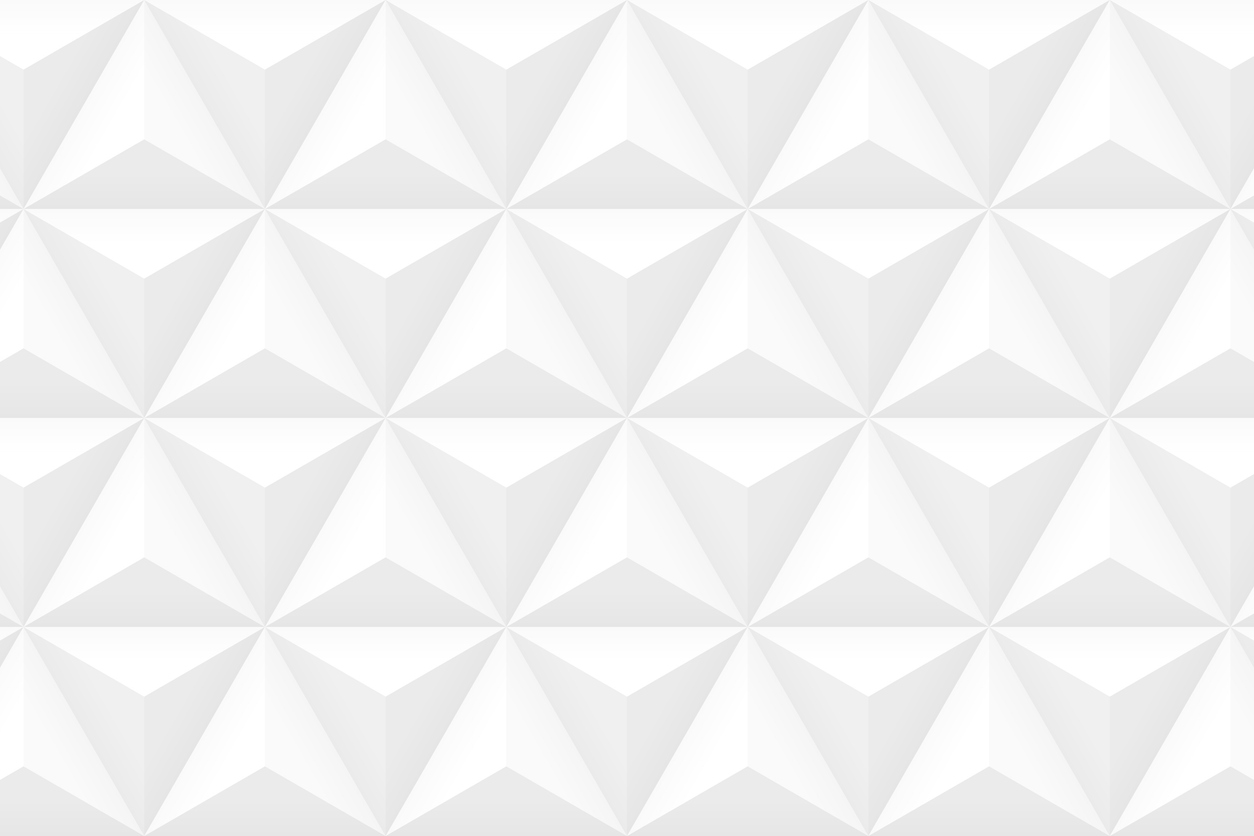
Simulations make Copenhagen the cycling capital
More than half of Copenhageners cycle every day. PTV Vissim’s microscopic simulations enable the city to realistically simulate and predict bicycle traffic and help redesign the infrastructure.
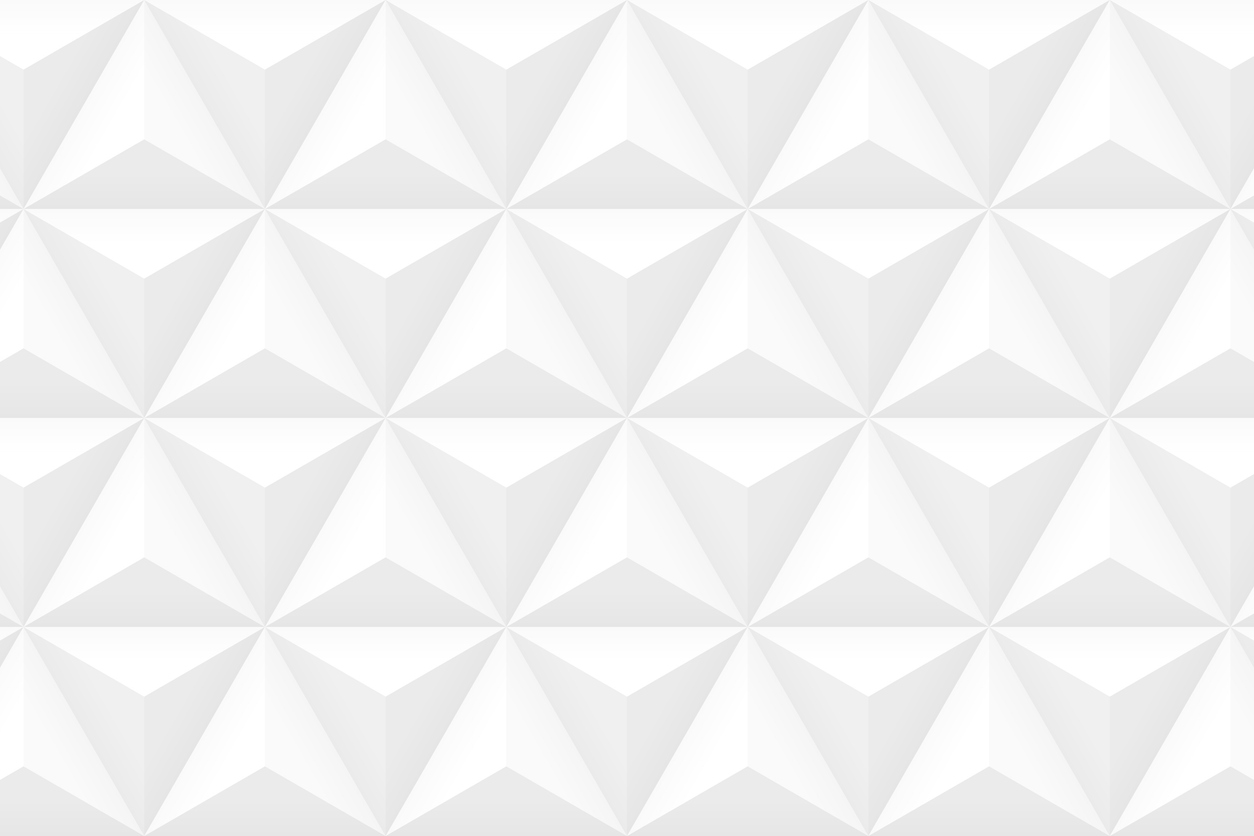
Get More People on Bikes!
A well-planned and safe infrastructure is key to increasing cycling. PTV Visum helps
analyze potential measures in advance.