For our April Fools’ joke, we envisioned what MobilityGPT – a kind of chatGPT for mobility – would look like: An AI-powered system that generates a transportation model for any city or region in the world as well as for any desired use case within seconds – just by typing in a description. Is there something to this idea or is it just a joke? We asked our CEO, Christian U. Haas, if and to what extent PTV already uses AI-technology.
Christian, how realistic is ModelGPT?
Christian: The story was over the top but has realistic aspects. First of all, the revolutionary and super-fast technology to automize the building of standardized transport models does already exist: With Model2Go, basic transportation models can be set up for any given city or region worldwide within just a few days – a process that takes months manually and requires lots off knowhow and resources.
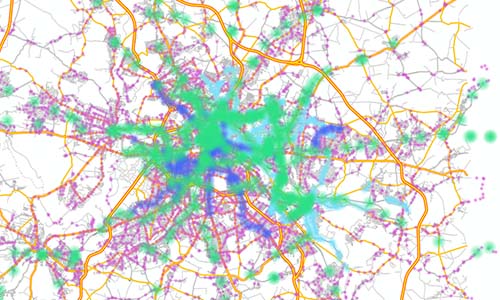
Since summer 2022, when we launched this automated technology, numerous customers are reaching out in order to try and work with it. What we learn is, that this technology brings a significant change to their work. It drastically reduces the time, effort, and cost of model-building, making model-based decisions viable even for small projects. Additionally, Model2Go is empowered by Machine Learning (ML) and Big Data sources. We are constantly developing Model2Go on this path. For example, structural data, jobs, inhabitants are also to be predicted with artificial intelligence, which will improve the results.
Is AI technology part of other PTV products too?
Christian: Absolutely. AI comes into play in numerous areas. We included it, e.g., in the field of recognizing and reconstructing the geographical distribution of travel demand. It also plays a major role for recognizing the mode of transport based on GPS data or trajectories. Depending on the data source, it can be hard to get details on the mode of travel. For mobile device location-based services (LBS) we can tell that people have moved from A to B, but not how. This information is crucial, however, e.g., in modeling changing travel behavior on sustainable modes, for example. AI classification methods help improve these valuable data sets by synthesizing missing and additional characteristics.
What about intelligent traffic management?
Christian: Another important application area for sure. Our real-time traffic management solution PTV Optima combines machine learning techniques with dynamic traffic modeling. The ML algorithms are used for forecasting calculations and improving the accuracy of the model. Today’s mobility systems are becoming increasingly complex with all the different transport modes, new technologies and services. To orchestrate the interaction, it is necessary to not only get a coherent and detailed picture of the current traffic situation, but also being able to act in a predictive way. Our software not only provides live information on the current traffic situation, but also enables detailed traffic forecasts up to 60 minutes in advance. This enables traffic managers to react appropriately to both planned and unplanned events.
Here, too, we are constantly working on further developments – for example, we are using ML to point out to traffic operators that a specific area might need more attention. Machine Learning is also part of the new and automated process that generates meaningful alerts aimed to prevent dangerously congested situations, therefore increasing safety and smooth operation of road networks.
In transit networks, we work on ML techniques to propose mitigation actions when service disruptions occur due to vehicle breakdowns, unexpected congestion, and similar situations.
Can you reveal some insights on projects, that base on AI …
Christian: We are part of the Mobility Data Space – the data sharing community brought to live by the German federal government. For them we developed a use case to enable short-term forecasts of traffic volumes on selected stretches of the highway network, which is based on a specifically developed machine learning system that analyzes historical traffic patterns and compares them with current traffic conditions. Often, traffic volumes are still forecasted statically, without taking current traffic patterns into account. For municipalities, traffic management service providers or other mobility providers, this use case and the ability to manage traffic and demand in real time can deliver great added value.
A very exciting project is #transmove in Hamburg. In close cooperation with the Free and Hanseatic City of Hamburg (namely the Department of Roads, Bridges and Waters), KIT Karlsruhe and the industrial partner Workplace Solutions GmbH (WPS) we are implementing an AI-supported software that aims to enable smart and sustainable mobility forecasts (short and long term) Hamburg strives to use the holistic approach not only to improve city-wide mobility, but also to reduce emissions. The exciting thing about it: The plan is to make this smart system available not only to mobility planners such as traffic control centers and thus to city/road/bike path planners, but also to citizens.
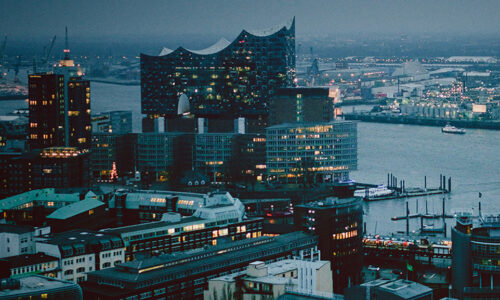